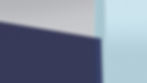
ABSTRACT
In this paper, we work towards linking users’ identities on different social media platforms by exploring the user generated contents (UGCs). This task is non-trivial due to the following challenges. 1) As UGCs involve multiple modalities (e.g., text and image), how to accurately characterize user accounts based on their heterogeneous multi-modal UGCs poses the main challenge. 2) As people tend to post similar UGCs on different social media platforms during the same period, how to effectively model the temporal post correlation is a crucial challenge. And 3) no public benchmark dataset is available to support user identity linkage based on heterogenous UGCs. Towards this end, we present an attentive time-aware user identity linkage scheme, which seamlessly integrates the temporal post correlation modeling and attentive user similarity modeling. To facilitate the evaluation, we construct a comprehensive large-scale user identity linkage dataset from two popular social media platforms: Instagram and Twitter. Extensive experiments have been conducted on our dataset, and the results verify the effectiveness of the proposed scheme. As a residual product, we have released our dataset, codes, and parameters to facilitate other researchers.
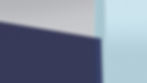
CHALLENGE
The user similarity modeling based on heterogeneous UGCs is nontrivial
due to the following reasons. 1) UGCs may involve multiple image and corresponding textual description in a UGC may have different confidences pertaining to the user characterization. Therefore, how to adaptively characterize the confidence of different modalities towards user identity linkage poses the main challenge for us. 2) In fact, one user tends to post similar, even the same UGCs across different social media during the same period. Consequently, how to incorporate the temporal post correlation between users’ distributed UGCs into the user similarity modeling is a tough challenge. And 3) there is no publicly available large-scale dataset to well support our user identity linkage task based on users’ heterogeneous UGCs. Therefore, the last challenge lies in the lack of benchmark datasets.
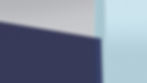
DATA
We construct a large-scale user identity linkage dataset, dubbed as TWIN,
comprising 5,765 users from Twitter and Instagram, respectively. (download)
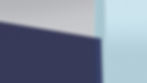
CODE
This is our proposed model for user identity linkage, which seamlessly integrates the temporal post correlation modeling and attentive user similarity modeling. (download)